New U.S chip rules could be very bad, for American and Chinese firms
New American restrictions on sales of high-end chips to China could profoundly impact semiconductor, cloud, and AI companies and technologies all over the world, from London to Taipei
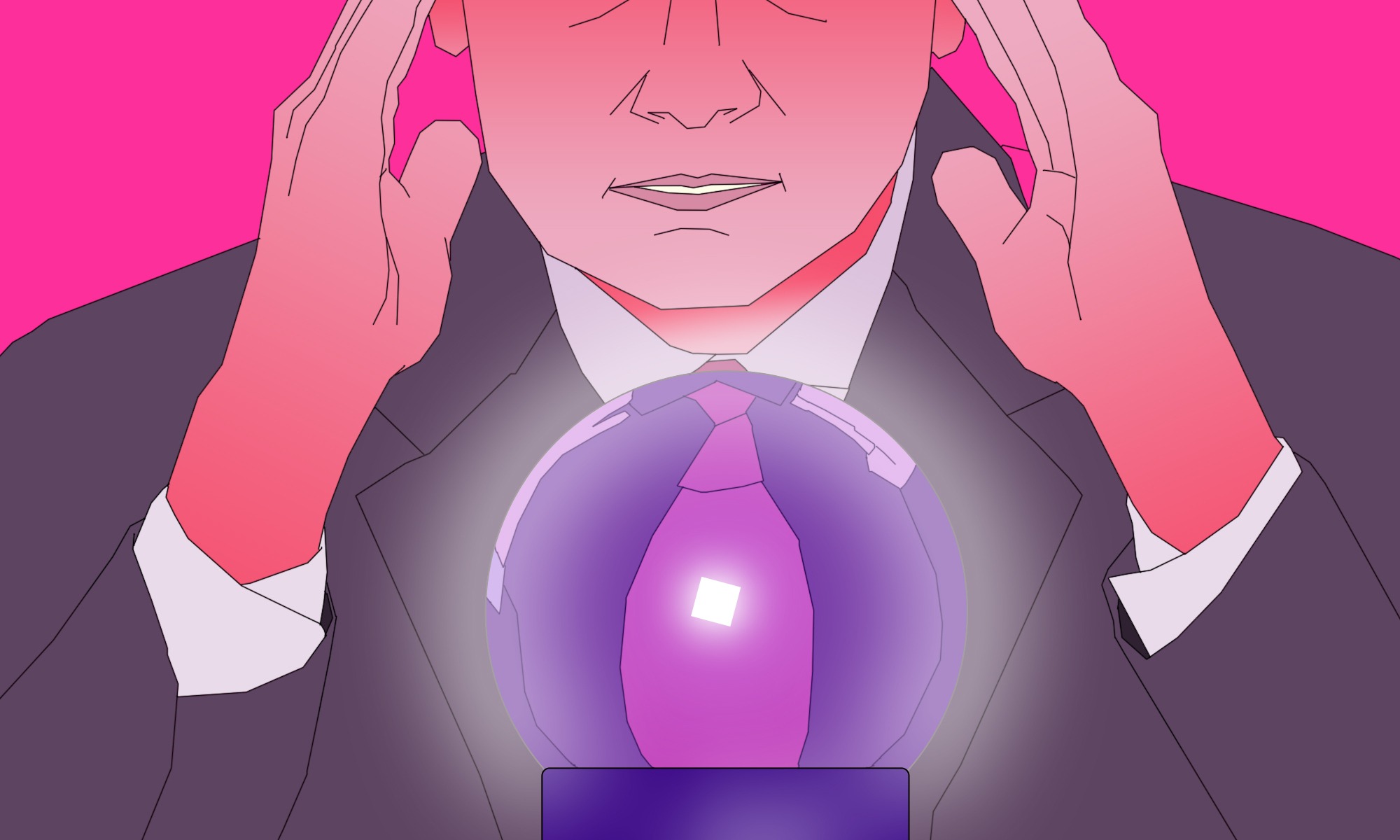
Last month, just as the dust had settled on the passage of the U.S. CHIPS and Science Act, with its guardrails targeting China and incentives designed to onshore advanced semiconductor manufacturing, yet another bombshell dropped in the U.S.-China Technology Cold War.
Nvidia and AMD, leading makers of advanced semiconductors used for artificial intelligence (AI) and small-scale high-performance computing (HPC) applications, were notified by the U.S. Commerce Department in August that new controls were coming that would impact sales of their high-end chips to Chinese end users. According to an SEC filing submitted by Nvidia, the company will be required to apply for a license when exporting its A100 series and upcoming H100 series graphics processing units (GPUs) to China or Russia. Sales of equipment containing those chips will also be restricted, as will “any future NVIDIA integrated circuit achieving [performance] roughly equivalent to the A100, as well as any system that includes those circuits.”
Nvidia’s stock tanked as the firm suggested that its quarterly revenue could take a hit of $400 million if Chinese buyers could not be persuaded to purchase alternative Nvidia products. Although it appears the ban will be phased in and not kick in completely until September 2023, the die has been cast.
AMD confirmed it had received a similar notice, and it is likely that other American chipmakers will also be impacted by the ban.
The new U.S. controls suggest that we are on the cusp of a brand new phase of U.S.-China tech competition. They also hint that the Biden Administration’s China containment strategy has broadened. The detailed rules governing the controls on GPUs are likely to be released by the Commerce Department soon, as early as this week. In addition, it is possible that the new rules will extend extraterritorial export controls under the foreign direct product rule to dozens of Chinese HPC and AI research centers.
Why is the Biden administration enacting these new rules now?
Longstanding concerns over China’s huge investments in supercomputing, more recent concerns over the country’s AI capabilities, and the growing power of smaller scale platforms for AI and HPC modeling appear to be behind the move. While China’s advances in AI hardware worry the U.S. government for any number of reasons, this particular ban appears to be driven by concern in national security circles — particularly the Department of Defense – about diversion of these systems to military end use.
Chinese supercomputers are rumored to have reached exascale data processing speeds. This means they have gigantic processing power (of at least one exaflop floating point calculation per second), making them capable of everything from weather modeling to simulating hypersonic glide vehicles. Given sensitivities about revealing China’s capabilities, Chinese officials appear to be limiting information release around performance and details about hardware. In addition, HPCs — including some designed by Chinese companies — are now being equipped with hardware accelerators, such as the graphics processing units (GPUs) made by Nvidia and AMD, and are being used to run AI workloads.
HPC systems and AI have been on the path toward convergence for some time, as the introduction of AI — particularly in the form of machine learning — promises to open new frontiers for supercomputing. Exascale machines will contain on the order of 135,000 GPUs and 50,000 CPUs, and each of those chips will have many individual processing units, requiring engineers to write programs that execute almost a billion instructions simultaneously. Already, of the HPCs on the Top 500 list of most powerful computers, more than 100 use Nvidia GPUs, including in China, primarily A100s and V100s, for acceleration and optimization of math intensive workloads.
These same GPUs, coupled with high end CPUs, and other semiconductors such as field programmable gate arrays (FPGAs), are all being used by U.S. and Chinese firms to run AI training algorithms in the cloud, along with application-specific integrated circuits (ASIC) designed to run specific types of AI algorithms, like the Tesla ASICs used for video processing to assist full self-driving and autopilot. Other ASICs from startups like the U.K.’s Graphcore and U.S. firm Cerebras are being designed and manufactured by advanced foundries and incorporated into large systems and used to optimize running of AI workloads.
This explosion in the development of semiconductors capable of being used to train and run AI algorithms and HPC workloads on smaller systems that could have military applications has led to the point where U.S. government officials now see the need to impose new controls on hardware and software around AI and HPC applications, including both large-scale systems and smaller clusters of GPUs that can be used as test algorithms more cheaply initially before running workloads on large systems. For example, last year’s Entity List action against Chinese CPU Phytium centered on the use of the firm’s CPUs in HPC systems likely used to model China’s hypersonic glide vehicle, a system that has raised alarm bells at the Pentagon because of its potential to evade advanced warning systems and carry nuclear weapons.
Beyond specific defense-related concerns, experts have spent the last five years raising alarms about China’s rising competitiveness in AI more broadly. In his book AI Superpowers, Kai-Fu Lee argues that China is positioned to become the global leader in AI, in part due to the massive amounts of data generated by the country’s huge mobile-connected population, abundant AI development talent, and dedicated state support for the industry. While competing countries such as the U.S. can’t reasonably hamper China’s ability to generate data and develop algorithms, or reduce the state’s enthusiasm for artificial intelligence, they can apply pressure to China’s ability to train those algorithms efficiently by restricting access to the necessary hardware.
All of this has paved the road to the latest Commerce Department bans.
How much will U.S. companies suffer?
Many industry advocates over the past several years have called for the US government to adopt a “small yard high fence” approach to controlling advanced technologies. In essence, that means keeping the number of advanced technologies that are restricted for access small, but placing heavy restrictions on those technologies that do make the list. This idea has been advocated by American commenters who see the economic benefits of large scale tech flows between the U.S. and China, and want to minimize the damage done to them by rules intended to protect American national security.
This latest ban certainly represents a challenge to this approach.
That said, the full scope of the new regulations being proposed remains unclear, for several reasons. First, it is not known how many U.S. chip companies received a similar notice. Second, the Commerce Department has not clarified if it is targeting only GPUs, or is taking a wider approach by restricting exports — or planning to restrict exports — of any advanced chips that can support AI-related processes. Such a list could grow to be extremely long, and could include a variety of CPUs, niche chips such as Google’s tensor processing units (TPUs), FPGAs, ASICS, and related technologies that can be used for running large HPC workloads and training AI algorithms. A restriction on Intel exports of advanced CPUs, for example, would represent a brutal blow to Chinese supercomputing hardware manufacturers.
Based on the contents of Nvidia’s SEC filing, it’s likely that the new U.S. controls will be based on some type of performance parameter related to these systems, such as processing power for a single chip or aggregate performance for a cluster of GPUs. Considering that technology is constantly in flux, this is a moving target. It also raises questions about whether or not such chips will be derestricted once they are no longer cutting edge, or whether such controls will remain in place even as technology advances.
Last but certainly not least, it is unclear how many Chinese devices require such chips, and how many and which Chinese companies currently import equipment that contains such chips.
What is certain is that the potential collateral effects of further restrictions on advanced chip sales to China are high — particularly for U.S. firms. In 2021, chip sales to China totaled $85.4 billion, with Qualcomm, Intel, and Texas Instruments taking in $22.5 billion, $21.1 billion, and $10 billion respectively. While it’s unclear what percentage of those sales are derived from advanced AI-friendly chips, a back-of-the-napkin estimate indicates that impacts could be significant. Nvidia’s 2021 China revenue stood at $7.1 billion, which means the company’s projected potential $400 million loss due to the recent sanction represents roughly 5.6% of its total China-derived revenue. If we rather cheekily extend that same potential loss to the industry as a whole, U.S. industry could lose out on $4.8 billion of revenue.
Additionally, U.S. firms would miss out on the considerable growth in the market for cloud-based semiconductors for training AI algorithms in China. The hardware portion of a typical AI system includes many different types of semiconductors: for example, one or more accelerators — CPUs, GPUs, FPGAs, or ASICs — which are designed to perform the highly parallel computations required by AI algorithms; short-term memory — typically dynamic random-access memory, or DRAM; and long-term storage-related memory — typically NAND. These devices are usually networked in the cloud, while operating either connected to a cloud-based system or independently on the edge. U.S. firms are strong producers of all of the above.
The U.S. is not the only player on the field, however. Newer designs such as the U.K. startup Graphcore’s Intelligence Processing Units (IPUs), for example, have been created specifically for AI workloads in the cloud. Graphcore claims its Colossus MK2 GC200 is designed to compete with Nvidia’s A100. Graphcore claims that a 16 IPU-Machine M2000 aimed at the data center market will achieve 16 petaFLOPS, sufficient to train the largest AI models using billions of parameters. It makes little sense to remove the U.S. from the playing field, and allow companies like Graphcore to step in.
U.S. officials are likely also discussing broader plurilateral controls on AI hardware with allies, but it remains unclear how far along these are. Eventually, once U.S. rules around GPU are finalized, the issue will be taken up again under the Wassenaar Agreement, a multilateral group that controls some dual use technologies with military applications. This is unlikely to happen until next year.
Huge potential impact on China’s AI semiconductors and data centers
While it’s likely that the Biden Administration implemented this ban primarily to limit the advancement of China’s HPC and AI capabilities, the implications for China could be much broader than the U.S. intended. Notably, Beijing has recently embarked on a massive effort to increase China’s national computing power by building a nationwide grid of interconnected data centers — the National Unified Computing Power Network (NUCPN). Policymakers hope the NUCPN will allow China to pool computing resources from across the country, with computing power distributed where it is most needed, vaguely like an electrical grid. Advanced GPUs and other AI-friendly chips will be needed to meet the needs of this impending infrastructure buildout, chips that China may no longer be able to source from the U.S.
But more critically, one of the key goals of the NUCPN is to improve the energy efficiency of each new data center built, with an eye towards balancing the energy demands of all this new digital infrastructure with China’s carbon neutrality goals. One key pathway to reducing data center energy expenditure is through the process of virtualization, running multiple virtual servers or “instances” on a single chip. GPUs such as the A100 are particularly suited to this task, and it remains unclear if alternative Chinese sources for GPUs can fully replace suppliers such as Nvidia and AMD. Both Alibaba Cloud and Baidu Cloud are big users of A100 GPUs as part of their cloud services offerings, and many other AI computing centers in China are heavily reliant on American origin GPUs. In addition, domestic server giant Inspur routinely highlights its use of A00 GPUs in its flagship products—the firm is reportedly actively talking to Nvidia about the evolving situation.
Can Chinese companies make the chips?
However, top Chinese GPU makers will certainly try to step up. The same week as the Nvidia and AMD letters became public, another Chinese firm, Biren, announced that it was shipping its latest GPUs. Biren includes a number of former Nvidia and AMD engineers and managers, and has moved quickly to develop its line of GPUs. Other Chinese firms including Axera, Vastai, and Enflame Technology announced GPU plans at the September World AI Conference (WAIC) in Shanghai.
While many new Chinese companies in the AI semiconductor space are focused on edge AI chips, a small number are focusing on competing in the cloud-based deep learning accelerator chip market with the likes of Nvidia and AMD. For example, Enflame, founded in 2018, offers its Deep Thinking Unit series for cloud-based learning. It has been using Global Foundries 12 nm FinFET process for this iteration of the technology. The CTO of one domestic GPU contender, Shanghai-based Illuvator, was quoted in early September after news of the proposed ban became public, that his firm’s GPUs lagged far behind Nvidia’s products and software ecosystem.
These firms will now undoubtedly see a burst of funding as Beijing funnels support to its homegrown chip hopefuls. This is already happening to some degree, but funding is only part of the problem.
Indeed, the GPU came is not just about hardware. Nvidia has a huge advantage over rivals because of its unified device architecture, which includes a support environment for parallel programming that has been widely adopted by developers and is a cost effective and relatively straightforward method for boosting the performance of computer-intensive workloads. Chinese firms that are serious about competing with Nvidia would need to develop an entirely new software support ecosystem and convince developers to use it, currently a tall order.
Finally, the new U.S. rules due out as early as this week focusing on advanced GPU and optimized AI chip technology are likely to be accompanied by other controls on Chinese firms that are using TSMC as a manufacturing platform. The 2021 Entity Listing of Phytium, and TSMC’s almost immediate cessation of business with the firm could be duplicated if U.S. officials place any of the many other Chinese CPU, GPU, and ASIC design companies using TSMC or other foundry services. In fact, it would be inconsistent to restrict sales of Nvidia and AMD semiconductors to China and allow Chinese firms to replace them in the market, and maintain access to TSMC to iterate designs using the most advanced nodes.
All of this means that TSMC and Taiwan are once again likely to be drawn into the U.S. China Tech Cold War, with all the risks that entails. Technology competition is clearly at the center of U.S. China relations, as Secretary of State Antony Blinken stressed in his May 2022 speech on U.S. China policy. And all things semiconductor related are now at the center of technology competition, along with AI and high performance computing.